Ag Tech Sunday - The Two Main Reasons Big Data is Good for the Farm
- By: "Farm Tender" News
- Ag Company News
- Jan 26, 2019
- 778 views
- Share
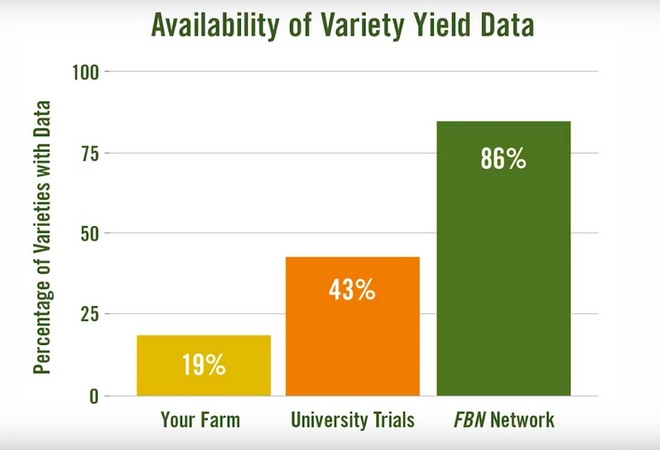
Although the concept of “big data” can sometimes be misunderstood, there are some major benefits to farmers using large datasets to make operational decisions.
Data-based insights (the patterns and trends that can be revealed by large datasets) have the potential to offer information that you can actually use for input decision-making.
1. Aggregating large datasets lets you learn and experiment faster.
Farmers use lots of different practices, plant many different varieties and experience diverse weather patterns that can change from year to year. By aggregating and analyzing data from a number of operations across those many variables, you can identify how all of these factors affect crop production.
Sure, you can run experiments on your own farm, but because there are many factors that affect yield, it would take decades to test all the possible factors on your own operation.
Let’s assume you farm 2,000 acres. To test 20 seed varieties, on 10 soil types, at 5 different seeding rates (and in order to analyze data on just 100 acres for each combination) would take 50 years—longer than the average farmer’s career, and much, much longer than we can wait to know the results.
Ad - For all you Hay and Grain Cartage call Bruce Robertson Transport - Australia wide - Ad
Ad - For all you Hay and Grain Cartage call Bruce Robertson Transport - Australia wide - Ad
By working together farmers have shared yields and we have analyzed them to give farmers more insights on production decisions.
Experimenting on your farm operation can be a risky, slow and expensive process. Leveraging existing data from other farms helps you learn, quickly, by taking advantage of the diversity of existing management practices.
Looking at networked data from FBN gives you access to data on many more varieties than if you only considered trial data from your own farm, or the varieties you’ve planted in the past.
what would you trust more?
2. Large datasets give you more confidence in results.
The important point about dataset size is that large datasets help to wash out the noise and errors that, in small datasets, can lead to misleading patterns.
For example, let’s say you plant two soybean varieties. Variety 1 performs better (higher yield) than Variety 2 - if this is based on only 1 acre of data, then it’s hard to know if Variety 1 is actually higher yielding, or if it just happened to, for example, experience favorable weather on that 1 acre. But if Variety 1 outperforms Variety 2 across 100,000 acres, then it’s unlikely that Variety 1 performed better just by chance.
With the small data set your farm alone can provide, even year over year, it can be hard to know if you’re observing a true pattern or just random variation. Large datasets cancel out the noise of smaller data sets and give you confidence that the patterns we observe are real.
Ad - For all you Hay and Grain Cartage call Bruce Robertson Transport - Australia wide - Ad
Ad - For all you Hay and Grain Cartage call Bruce Robertson Transport - Australia wide - Ad
If you are able to tell that a variety performed well across diverse growing conditions and on a number of acres—beyond a small trial plot—then you can have greater confidence in its performance. If you’re only looking at local data from similar farms, you could be in trouble if the conditions change.
Large datasets show that as more data is entered, the results are more accurate!
One question we often get is about any “noise” in the data, or the possibility of outliers in miscalibrated yield monitors. When we get that question, it is because farmers want to know if these types of miscalibration impact data and can interrupt patterns and trends—they want to know if they can trust the data.
To answer the farmer’s question about how a miscalibrated yield monitor impacts the data:
In a small data set, a miscalibration could have a big impact. However, in a large dataset, those miscalibrations cancel themselves out. Some monitors record yields that are too low, and others record yields that are too high, but as long as these are occurring randomly, with enough data the average will not be significantly affected.
Calibrating yields after your harvest season can help your own farm's analytics but as an aggregate the network self-corrects itself
Ad - For all you Hay and Grain Cartage call Bruce Robertson Transport - Australia wide - Ad
Ad - For all you Hay and Grain Cartage call Bruce Robertson Transport - Australia wide - Ad
That’s why decisions based on aggregated data from larger datasets can help to remove bias. Insights from independent and unbiased data means that farmers can make decisions more confidently.
Remember: You should always calibrate your yield monitors for accurate benchmarking and better insights on your own operation. We also suggest network members submit their weigh tickets from a grain sale to post-calibrate yields and ensure accurate data.
https://emergence.fbn.com
https://emergence.fbn.com
Share Ag News Via